RELATED PROJECTS
CASE STUDIES
GET THE EBOOK
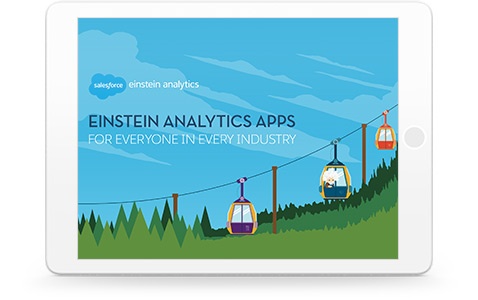
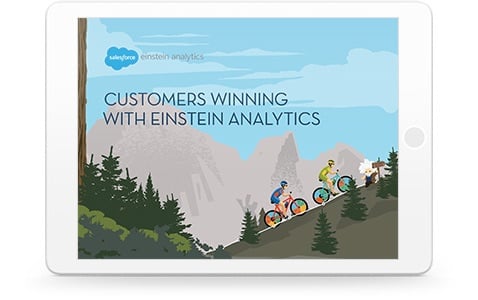
Use our B2B predictive analysis tool to Identify, engage and convert more customers effectively.
B2B Predictable Prospecting
When looking at the various B2B sales pipeline stages for a prospect to become and remain a customer, it’s clear that marketers need to be able to:
The backbone of all of this is data analytics: Data, coming from a variety of sources, are continuously captured, cleansed and consolidated. Mining techniques are then applied to find patterns and build appropriate models. Data analytics may be predictive (based in machine learning techniques) when voluminous historical data are available or descriptive, producing an easy, quick and indicative conclusion.
Search and discovery tools allow marketers to extract information from large unstructured databases. Unstructured data often have a higher level of ambiguity and higher difficulties in terms of integration and analysis. Improving the performance of this data source will improve accuracy in B2B user intent data, and then, subsequently, better prediction accuracy.
Many B2B companies are faced with the challenge of multiple and distributed sales and marketing systems and applications, each with its own silo of data. Integration of customer data is critical. If data from various sources—external or internal—can be effectively correlated and analyzed, prediction accuracies will improve.
Software systems that combine the predictive engine with analytics and transactional processing are the industry leaders. Enterprise-level CRM systems like the Salesforce platform, powered by the Einstein AI engine and with integration capabilities and strong analytics, fulfill all predictive analytics activities. Its tier-based architecture allows separation of analysis infrastructure with tools that may use its output. Learn more on Salesforce Predictive MarTech Alignment.
B2B predictable prospecting, using machine-learning techniques, is the most widely used application of artificial intelligence. It has proven to be a highly effective and popular tool for marketers, as demonstrated by the year-over-year doubling of implementations and the expectation for investments to quadruple in 2021 compared to 2017. However, with a wide and relatively non-uniform prospect pool, and visitor anonymity, predictive analysis (analysis of historical data) can only go so far in the B2B marketplace. User intent data analysis can fill the gap. Using predictable prospecting—user intent data paired with predictive analysis—marketers can more effectively identify, engage with and convert prospects. Although figures show a significant increase in area of application and investment, there is room for improvement in 2019 in performance and accuracy and in expanding application in other areas.
As a new Salesforce customer, it has been difficult for us to get reliable, smart consultants/programmers. Mark and his team were exactly what we needed. They communicated exceptionally well and worked with us to fully understand our needs. I look forward to working with Cetrix on future projects.
President, Aquasol & Poolsure
Mark is great to work with ... He did a great job describing the solution in the proposal, was available when we needed him ... He went above and beyond to help complete our proposal on a very tight timeline.
Founder & CEO, Acutedge, Inc.
Cetrix was easy to work with, worked quickly, and the work was excellent. Going into the job I didn't know exactly how to talk about what it was that our organization needed done with Salesforce, but they were able to easily figure out what our needs were and customize Salesforce for us. We were very pleased with their work.
The Diversity Center of Northern Ohio
Our team had never used a CRM system, but people at Cetrix walked us through every step as patiently as they could. What I really loved was that they were able to add valuable suggestions to enhance our CRM site ... Cetix has truly been one of my best experiences, and we now consider them as part of the team ... Because of Cetrix, our team can better communicate with our clients.
Director, HBCU Tours
Mark was great! I couldn't ask for a better experience. Wait until you hear him on Skype; he is focused, patient and just the right combo of get'er done/calm. His written communication skills are outstanding. I'm looking forward to doing more work with Cetrix.
ScriptEd had the pleasure of working with Cetrix Cloud Services on a Salesforce Database Customization project ... the donation of services has allowed ScriptEd to efficiently and affordably build out the Salesforce CRM that we use to manage our programming ... Over the duration of the project, they were professional, patient, flexible, and thorough. ScriptEd is thankful for and would highly recommend the pro-bono services of Cetrix Cloud Services.
ScriptEd INC
© 2023 Cetdigit Cloud Services. All rights reserved.